第四次工业革命的基础将在很大程度上取决于数据(Data)和连接(Connectivity)性。能够开发或创建数据挖掘解决方案的分析服务(Analysis Services)将在这方面发挥关键作用。它可以帮助分析和预测针对潜在买家的客户购买行为的结果。(customer purchasing behavior)数据(Data)将成为一种新的自然资源,从这些未分类的数据中提取相关信息的过程将变得非常重要。因此,正确理解数据挖掘这个(Data Mining)术语(term –) 、它的过程和应用可以帮助我们为这个流行语开发一个整体的方法。
数据挖掘基础(Data Mining Basics)及其技术
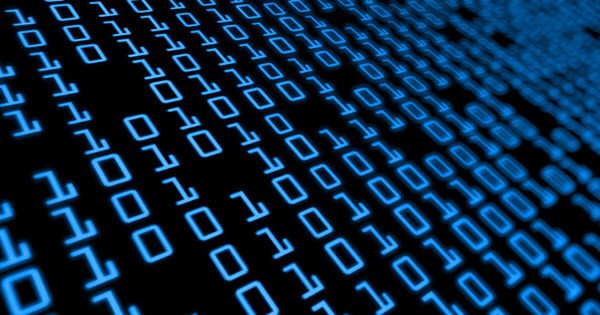
数据挖掘,也称为数据中的知识发现(Knowledge Discovery in Data)( KDD ),是关于搜索大型数据存储以发现超出简单分析的模式和趋势。然而,这不是一个单一步骤的解决方案,而是一个多步骤的过程,并且在不同的阶段完成。这些包括:
1] 数据收集和准备
它从数据收集及其适当的组织开始。这有助于显着提高找到可以通过数据挖掘发现的信息的机会
2] 模型构建和评估
数据挖掘过程(mining process)的第二步是各种建模技术的应用。这些用于将参数校准为最佳值。所采用的技术在很大程度上取决于满足各种组织需求和做出决策所需的分析能力。
让我们简要地研究一些数据挖掘技术。发现大多数组织将两种或多种数据挖掘技术结合在一起,形成满足其业务需求的适当流程。
阅读(Read):什么是大数据?
数据挖掘技术
- 关联—— (Association – )关联(Association)是广为人知的数据挖掘技术之一。在此基础上,基于同一事务中项目之间的关系破译模式。因此(Hence),它也被称为关系技术(relation technique)。大品牌零售商依靠这种技术来研究客户的购买习惯/偏好。例如,在跟踪人们的购买习惯时,零售商可能会发现顾客在购买巧克力时总是会购买奶油,因此建议他们下次购买巧克力时也可能想购买奶油。
- 分类(Classification)——这种数据挖掘技术(data mining technique)与上述不同之处在于它基于机器学习(machine learning)并使用线性规划(Linear programming)、决策树(Decision)、神经网络(Neural network)等数学技术。在分类中,公司尝试构建可以学习如何将数据项分类的软件。例如,一家公司可以在应用程序中定义一个分类,“给定所有提出从公司辞职的员工的记录,预测未来可能从公司辞职的人数。” 在这种情况下,公司可以将员工的记录分为“离开”和“留下”两类。然后它可以使用它的数据挖掘软件(mining software)将员工分类为之前创建的不同组。
- 集群(Clustering)——表现出相似特征的不同(Different)对象通过自动化组合在一个集群中。许多这样的集群被创建为类和对象(具有相似的特征)相应地放置在其中。为了更好地理解这一点,让我们考虑一个图书馆图书管理(book management)的例子。在图书馆中,大量的书籍被完全编目。相同类型的项目一起列出。这使我们更容易找到我们感兴趣的书。同样,通过使用聚类技术(clustering technique),我们可以将具有某种相似性的书籍保存在一个聚类中,并为其分配合适的名称。所以,如果读者想找一本相关的书(book relevant)对他的兴趣,他只需要去那个书架,而不是搜索整个图书馆。因此,聚类技术(clustering technique)定义类并将对象放入每个类中,而在分类技术中,对象被分配到预定义的类中。
- 预测(Prediction)——预测是一种数据挖掘技术(data mining technique),通常与其他数据挖掘技术(data mining technique)结合使用。它涉及分析趋势、分类、模式匹配(pattern matching)和关系。通过以适当的顺序(sequence one)分析过去的事件或实例,可以安全地预测未来的事件。例如,如果选择销售作为自变量,而利润(variable and profit)作为依赖于销售的变量,则预测分析技术(prediction analysis technique)可用于销售预测未来利润。然后,根据历史销售和利润数据(sale and profit data),可以绘制一条拟合回归曲线(regression curve),用于利润预测(profit prediction)。
- 决策树(Decision trees)——在决策树(decision tree)中,我们从一个有多个答案的简单问题开始。每个答案都会导致另一个问题,以帮助对数据进行分类或识别,以便对其进行分类,或者可以根据每个答案进行预测。例如,我们使用以下决策树(decision tree)来确定是否打板球ODI:数据挖掘决策树(Data Mining Decision Tree):从根节点(root node)开始,如果天气预报(weather forecast)预测下雨,我们应该避免当天的比赛。或者,如果天气预报(weather forecast)很清楚,我们应该打比赛。
数据挖掘(Data Mining)是各种行业和学科(如通信、保险(Insurance)、教育(Education)、制造(Manufacturing)、银行和零售(Banking and Retail)等)分析工作的核心。因此,在应用不同的技术之前,有关于它的正确信息是必不可少的。
What is Data Mining? Basics and its Techniques.
The foundation of the fourth industrial revolution will largely depеnd upon Data and Connectivity. Analysis Services capable of developing or creating data mining solutions will play a key role in this regard. It could assist in analyzing and predicting outcomes of customer purchasing behavior for targeting potential buyers. Data will become a new natural resource and the process of extracting relevant information from this unsorted data will assume immense importance. As such, a proper understanding of the term – Data Mining, its processes, and application could help us in developing a holistic approach to this buzzword.
Data Mining Basics and its Techniques
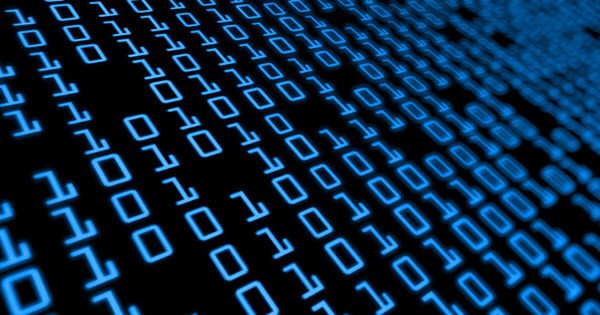
Data mining, also known as Knowledge Discovery in Data (KDD) is about searching large stores of data to uncover patterns and trends that go beyond simple analysis. This, however, is not a single-step solution but a multi-step process and is completed in various stages. These include:
1] Data gathering and Preparation
It starts with data collection and its proper organization. This helps in significantly improving the chances of finding the information that can be discovered through data mining
2] Model Building and Evaluation
The second step in data mining process is the application of various modeling techniques. These are used to calibrate the parameters to optimal values. Techniques employed largely depend on analytic capabilities required to address a gamut of organizational needs and to arrive at a decision.
Let us examine some data mining techniques in brief. It is found that most organizations combine two or more data mining techniques together to form an appropriate process that meets their business requirements.
Read: What is Big Data?
Data Mining Techniques
- Association – Association is one of the widely-known data mining techniques. Under this, a pattern is deciphered based on a relationship between items in the same transaction. Hence, it is also known as the relation technique. Big brand retailers rely on this technique to research customer’s buying habits/preferences. For example, when tracking people’s buying habits, retailers might identify that a customer always buys cream when they buy chocolates, and therefore suggest that the next time that they buy chocolates they might also want to buy cream.
- Classification – This data mining technique differs from the above in the way that it is based on machine learning and uses mathematical techniques such as Linear programming, Decision trees, Neural network. In classification, companies try to build software that can learn how to classify the data items into groups. For instance, a company can define a classification in the application that “given all records of employees who offered to resign from the company, predict the number of individuals who are likely to resign from the company in future.” Under such a scenario, the company can classify the records of employees into two groups that namely “leave” and “stay”. It can then use its data mining software to classify the employees into separate groups created earlier.
- Clustering – Different objects exhibiting similar characteristics are grouped together in a single cluster via automation. Many such clusters are created as classes and objects (with similar characteristics) are placed in it accordingly. To understand this better, let us consider an example of book management in the library. In a library, the vast collection of books is fully cataloged. Items of the same type are listed together. This makes it easier for us to find a book of our interest. Similarly, by using the clustering technique, we can keep books that have some kinds of similarities in one cluster and assign it a suitable name. So, if a reader is looking to grab a book relevant to his interest, he only has to go to that shelf instead of searching the entire library. Thus, the clustering technique defines the classes and puts objects in each class, while in the classification techniques, objects are assigned into predefined classes.
- Prediction – The prediction is a data mining technique that is often used in combination with the other data mining techniques. It involves analyzing trends, classification, pattern matching, and relation. By analyzing past events or instances in a proper sequence one can safely predict a future event. For instance, the prediction analysis technique can be used in the sale to predict future profit if the sale is chosen as an independent variable and profit as a variable dependent on sale. Then, based on the historical sale and profit data, one can draw a fitted regression curve that is used for profit prediction.
- Decision trees – Within the decision tree, we start with a simple question that has multiple answers. Each answer leads to a further question to help classify or identify the data so that it can be categorized, or so that a prediction can be made based on each answer. For example, We use the following decision tree to determine whether or not to play cricket ODI: Data Mining Decision Tree: Starting at the root node, if the weather forecast predicts rain then, we should avoid the match for the day. Alternatively, if the weather forecast is clear, we should play the match.
Data Mining is at the heart of analytics efforts across a variety of industries and disciplines like communications, Insurance, Education, Manufacturing, Banking and Retail and more. Therefore, having correct information about it is essential before apply the different techniques.