连接到Internet的设备称为智能设备。几乎所有与互联网(Internet)相关的东西都被称为智能设备(smart device)。在这种情况下,使设备更智能的代码——(SMARTER – )因此它可以在最少或没有任何人为干预的情况下工作——可以说是基于人工智能(Artificial Intelligence)(AI)。另外两个,即:机器学习(Machine Learning)(ML) 和深度学习(Deep Learning)(DL),是为智能设备带来更多功能而构建的不同类型的算法。让我们在下面详细了解AI、ML 和 DL(AI vs ML vs DL ),以了解它们的作用以及它们如何与 AI 连接。
什么是关于 ML 和 DL 的人工智能
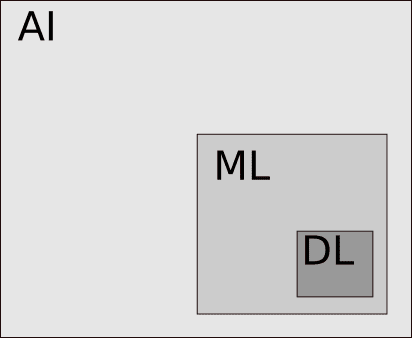
AI 可以称为机器学习(Machine Learning)(ML) 过程和深度学习(Deep Learning)(DL) 过程的超集。AI 通常是用于 ML 和 DL 的总称。深度学习又是(Deep Learning)机器学习(Machine Learning)的一个子集(见上图)。
一些人认为机器学习(Machine Learning)不再是通用人工智能的一部分。他们说 ML 本身就是一门完整的科学,因此不必参考人工智能(Artificial Intelligence)来称呼它。人工智能在数据上蓬勃发展:大数据(Big Data)。它消耗的数据越多,它就越准确。并不是说它总是会正确预测。也会有错误的标志。人工智能会针对这些错误进行自我训练,并在它应该做的事情上变得更好——无论是否有人监督。
人工智能无法正确定义,因为它已经渗透到几乎所有行业并影响太多类型的(业务)流程和算法。可以说,人工智能基于(Intelligence)数据科学(Data Science)(DS:Big Data),并包含机器学习(Machine Learning)作为其独特的部分。同样(Likewise),深度学习(Deep Learning)是机器学习(Machine Learning)的一个独特部分。
IT 市场正在倾斜,未来将由连接的智能设备主导,称为物联网 (IoT)(Internet of Things (IoT))。智能(Smart)设备意味着人工智能:直接或间接。您已经在日常生活中的许多任务中使用人工智能 (AI)。例如,在智能手机键盘上打字,“单词建议”会越来越好。在您不知不觉中与人工智能打交道的其他示例包括在(Artificial Intelligence)Internet上搜索内容、在线购物,当然还有智能的Gmail和Outlook电子邮件收件箱。
什么是机器学习
机器学习(Learning)是人工智能(Artificial Intelligence)的一个领域,其目的是让机器(或计算机,或软件)在没有太多编程的情况下学习和训练自己。这些设备需要较少的编程,因为它们应用人类方法来完成任务,包括学习如何更好地执行。基本上(Basically),ML 意味着对计算机/设备/软件进行一些编程并允许其自行学习。
有几种方法可以促进机器学习(Machine Learning)。其中,以下三种被广泛使用:
- 监督,
- 无人监督,和
- 强化学习。
机器学习(Machine Learning)中的监督学习
监督在某种意义上,程序员首先向机器提供标记数据和已经处理的答案。在这里,标签是指数据库或电子表格中的行名或列名。在将大量此类数据输入计算机后,它就可以分析进一步的数据集并自行提供结果。这意味着您教计算机如何分析输入给它的数据。
通常,使用 80/20 规则来确认。大量(Huge)数据被输入计算机,该计算机尝试并学习答案背后的逻辑。80% 的事件数据连同答案一起输入计算机。剩下的 20% 没有答案,看计算机是否能得出正确的结果。这 20% 用于交叉检查以了解计算机(机器)是如何学习的。
无监督机器学习
无监督学习发生在机器被提供没有标记且不按顺序的随机数据集时。机器必须弄清楚如何产生结果。例如,如果你给它提供不同颜色的垒球,它应该能够按颜色分类。因此,将来,当机器收到一个新的垒球时,它可以在其数据库中识别具有已存在标签的球。这种方法没有训练数据。机器必须自己学习。
强化学习
可以做出一系列决策的机器属于这一类。然后是奖励制度。如果机器在程序员想要的任何事情上做得很好,它就会得到奖励。机器的编程方式使其渴望获得最大的回报。为了得到它,它通过在不同情况下设计不同的算法来解决问题。这意味着人工智能计算机使用试错法来得出结果。
例如,如果机器是自动驾驶汽车,它必须在道路上创建自己的场景。程序员不可能对每一步都进行编程,因为当机器在路上时,他或她无法想到所有的可能性。这就是强化学习(Reinforcement Learning)的用武之地。你也可以称之为试错法人工智能。
深度学习与机器学习有何不同(Machine Learning)
深度学习(Deep Learning)适用于更复杂的任务。深度学习(Deep Learning)是机器学习(Machine Learning)的一个子集。只是它包含更多帮助机器学习的神经网络。人造(Manmade)神经网络并不新鲜。世界各地的实验室(Labs)都在尝试构建和改进神经网络,以便机器能够做出明智的决定。你一定听说过Sophia ,一个在(Sophia)沙特(Saudi)获得普通公民身份的人形机器人。神经网络就像人类的大脑,但没有大脑那么复杂。
有一些很好的网络可以提供无监督深度学习。你可以说深度学习(Deep Learning)更多的是模仿人脑的神经网络。尽管如此,有了足够的样本数据,深度学习(Deep Learning)算法仍可用于从样本数据中提取细节。例如,使用图像处理器 DL 机器,更容易根据机器提出的问题创建情绪变化的人脸。
上面用更简单的语言解释了 AI、MI 和 DL。AI 和 ML 是广阔的领域——它们刚刚开放并且具有巨大的潜力。这就是一些人反对在人工智能中使用(Artificial Intelligence)机器学习(Machine Learning)和深度学习(Deep Learning)的原因。
What are Machine Learning and Deep Learning in Artificial Intelligence
Dеvices connected tо the Internet are called smart devices. Pretty much everything related to the Internet is known aѕ a smart device. In this context, the code that makes the devices SMARTER – so that it can work with minimal or without any human intervention – can be said to be based on Artificial Intelligence (AI). The other two, namely: Machine Learning (ML), and Deep Learning (DL), are different types of algorithms built to bring more capabilities to the smart devices. Let’s see AI vs ML vs DL in detail below to understand what they do and how they are connected to AI.
What is Artificial Intelligence with respect to ML & DL
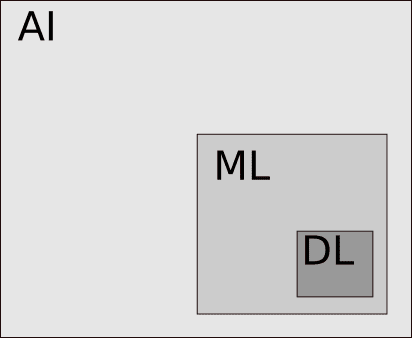
AI can be called a superset of Machine Learning (ML) processes, and Deep Learning (DL) processes. AI usually is an umbrella term that is used for ML and DL. Deep Learning is again, a subset of Machine Learning (see image above).
Some argue that Machine Learning is no more a part of the universal AI. They say ML is a complete science in its own right and thus, need not be called with reference to Artificial Intelligence. AI thrives on data: Big Data. The more data it consumes, the more accurate it is. It is not that it will always predict correctly. There will be false flags as well. The AI trains itself on these mistakes and becomes better at what it is supposed to do – with or without human supervision.
Artificial Intelligence cannot be defined properly as it has penetrated into almost all industries and affects way too many types of (business) processes and algorithms. We can say that Artificial Intelligence is based on Data Science (DS: Big Data) and contains Machine Learning as its distinct part. Likewise, Deep Learning is a distinct part of Machine Learning.
The way the IT market is tilting, the future would be dominated with connected smart devices, called the Internet of Things (IoT). Smart devices mean artificial intelligence: directly or indirectly. You are already using artificial intelligence (AI) in many tasks in your daily life. For example, typing on a smartphone keyboard that keeps on getting better on “words suggestion”. Among other examples where you unknowingly are dealing with Artificial Intelligence are searching for things on the Internet, online shopping, and of course, the ever-smart Gmail and Outlook email inboxes.
What is Machine Learning
Machine Learning is a field of Artificial Intelligence where the aim is to make a machine (or computer, or a software) learn and train itself without much programming. Such devices need less programming as they apply human methods to complete tasks, including learning how to perform better. Basically, ML means programming a computer/device/software a bit and allowing it to learn on its own.
There are several methods to facilitate Machine Learning. Of them, the following three are used extensively:
- Supervised,
- Unsupervised, and
- Reinforcement learning.
Supervised Learning in Machine Learning
Supervised in a sense that programmers first provide the machine with labeled data and already processed answers. Here, labels mean the row or column names in a database or spreadsheet. After feeding huge sets of such data to the computer, it is ready to analyze further data sets and provide results on its own. That means you taught the computer how to analyze the data fed to it.
Usually, it is confirmed using the 80/20 rule. Huge sets of data are fed to a computer that tries and learns the logic behind the answers. 80 percent of data from an event is fed to the computer along with answers. The remaining 20 percent is fed without answers to see if the computer can come up with proper results. This 20 percent is used for cross-checking to see how the computer (machine) is learning.
Unsupervised Machine Learning
Unsupervised Learning happens when the machine is fed with random data sets that are not labeled, and not in order. The machine has to figure out how to produce the results. For example, if you offer it softballs of different colors, it should be able to categorize by colors. Thus, in the future, when the machine is presented with a new softball, it can identify the ball with already present labels in its database. There is no training data in this method. The machine has to learn on its own.
Reinforcement Learning
Machines that can make a sequence of decisions fall into this category. Then there is a reward system. If the machine does good at whatever the programmer wants, it gets a reward. The machine is programmed in a way that it craves maximum rewards. And to get it, it solves problems by devising different algorithms in different cases. That means the AI computer uses trial and error methods to come up with results.
For example, if the machine is a self-driving vehicle, it has to create its own scenarios on road. There is no way a programmer can program every step as he or she can’t think of all the possibilities when the machine is on the road. That is where Reinforcement Learning comes in. You can also call it trial and error AI.
How is Deep Learning different from Machine Learning
Deep Learning is for more complicated tasks. Deep Learning is a subset of Machine Learning. Only that it contains more neural networks that help the machine in learning. Manmade neural networks are not new. Labs across the world are trying to build and improve neural networks so that the machines can make informed decisions. You must have heard of Sophia, a humanoid in Saudi that was provided regular citizenship. Neural networks are like human brains but not as sophisticated as the brain.
There are some good networks that provide for unsupervised deep learning. You can say that Deep Learning is more neural networks that imitate the human brain. Still, with enough sample data, the Deep Learning algorithms can be used to pick up details from sample data. For example, with an image processor DL machine, it is easier to create human faces with emotions changing according to the questions the machine is asked.
The above explains AI vs MI vs DL in easier language. AI and ML are vast fields – that are just opening up and have tremendous potential. This is the reason some people are against using Machine Learning and Deep Learning in Artificial Intelligence.